Input
Configure model parameters
Output
View generated results
Result
Preview, share or download your results with a single click.
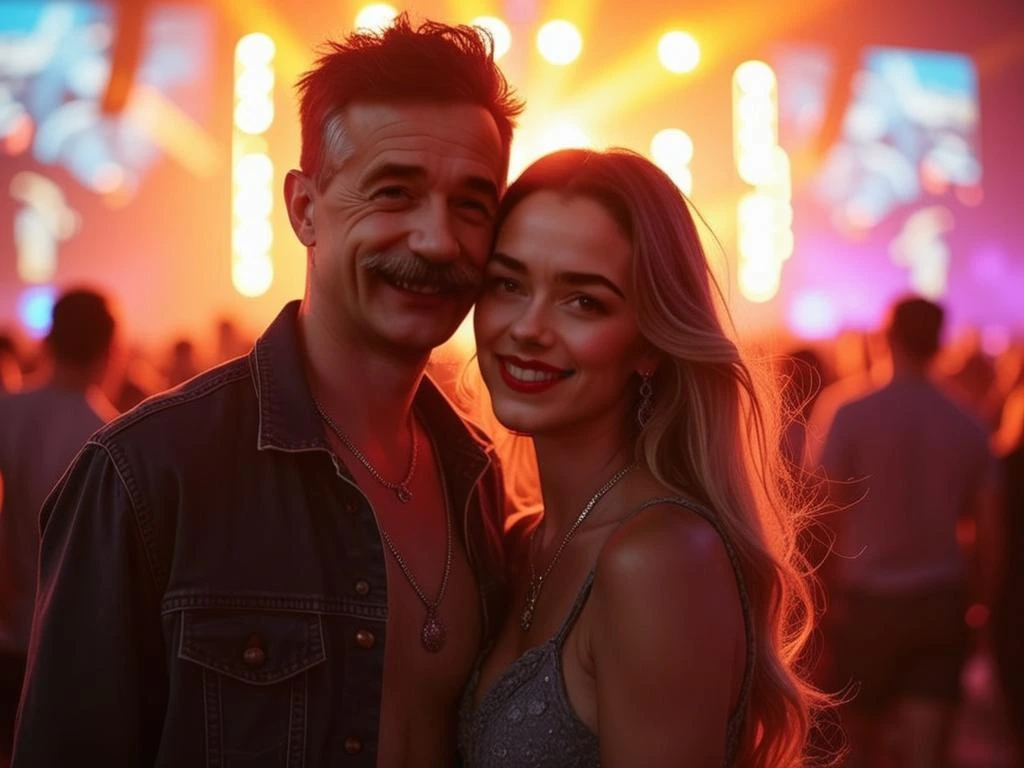
Prerequisites
- Create an API Key from the Eachlabs Console
- Install the required dependencies for your chosen language (e.g., requests for Python)
API Integration Steps
1. Create a Prediction
Send a POST request to create a new prediction. This will return a prediction ID that you'll use to check the result. The request should include your model inputs and API key.
import requestsimport timeAPI_KEY = "YOUR_API_KEY" # Replace with your API keyHEADERS = {"X-API-Key": API_KEY,"Content-Type": "application/json"}def create_prediction():response = requests.post("https://api.eachlabs.ai/v1/prediction/",headers=HEADERS,json={"model": "omni-zero-couples","version": "0.0.1","input": {"seed": -1,"prompt": "Cinematic still photo of a couple. emotional, harmonious, vignette, 4k epic detailed, shot on kodak, 35mm photo, sharp focus, high budget, cinemascope, moody, epic, gorgeous, film grain, grainy","base_image": "your_file.image/jpeg","depth_image": "your_file.image/jpeg","style_image": "your_file.image/jpeg","guidance_scale": 3,"negative_prompt": "anime, cartoon, graphic, (blur, blurry, bokeh), text, painting, crayon, graphite, abstract, glitch, deformed, mutated, ugly, disfigured","number_of_steps": 10,"identity_image_1": "your_file.image/jpeg","identity_image_2": "your_file.image/jpeg","number_of_images": 1,"mask_guidance_end": 1,"base_image_strength": 0.2,"mask_guidance_start": 0,"depth_image_strength": 0.2,"style_image_strength": 1,"identity_image_strength_1": 1,"identity_image_strength_2": 1},"webhook_url": ""})prediction = response.json()if prediction["status"] != "success":raise Exception(f"Prediction failed: {prediction}")return prediction["predictionID"]
2. Get Prediction Result
Poll the prediction endpoint with the prediction ID until the result is ready. The API uses long-polling, so you'll need to repeatedly check until you receive a success status.
def get_prediction(prediction_id):while True:result = requests.get(f"https://api.eachlabs.ai/v1/prediction/{prediction_id}",headers=HEADERS).json()if result["status"] == "success":return resultelif result["status"] == "error":raise Exception(f"Prediction failed: {result}")time.sleep(1) # Wait before polling again
3. Complete Example
Here's a complete example that puts it all together, including error handling and result processing. This shows how to create a prediction and wait for the result in a production environment.
try:# Create predictionprediction_id = create_prediction()print(f"Prediction created: {prediction_id}")# Get resultresult = get_prediction(prediction_id)print(f"Output URL: {result['output']}")print(f"Processing time: {result['metrics']['predict_time']}s")except Exception as e:print(f"Error: {e}")
Additional Information
- The API uses a two-step process: create prediction and poll for results
- Response time: ~12 seconds
- Rate limit: 60 requests/minute
- Concurrent requests: 10 maximum
- Use long-polling to check prediction status until completion
Overview
Omni-Zero-Couples is a diffusion-based text-to-image model that enables the creation of high-quality, stylized portraits of couples without the need for prior training on specific data. By leveraging advanced diffusion techniques, it allows for seamless blending of styles and identities, facilitating the generation of unique and personalized couple portraits with minimal effort. This model builds upon the foundation of the Omni-Zero model, which focused on single-subject portraits, expanding its capabilities to accommodate couples.
Technical Specifications
Model Architecture:
- Built on diffusion-based techniques optimized for style and subject blending.
- Fine-tuned for zero-shot generalization across diverse artistic genres.
Training Data:
- Trained on a diverse dataset containing a mix of portrait, artistic, and stylized images, ensuring wide applicability.
Key Considerations
Input Quality: High-resolution and relevant images enhance output quality.
Processing Time: Complex prompts and higher step counts may increase generation time.
Tips & Tricks
Style Exploration: Use various style images to create portraits in different artistic genres, such as Impressionism or Pop Art.
Identity Blending: Combine features from multiple identity images to produce composite portraits.
Negative Prompts: Specify elements to avoid (e.g., "no background clutter") to refine outputs.
Detailed Prompts: Use clear and precise language in prompts for more accurate results. For example, instead of "a couple in love," try "a romantic couple in a winter forest, Impressionist art style."
Experiment with Parameters: Adjust parameters like guidance scale, image strength, and number of steps to refine outputs.
High-Quality Inputs: If you use reference images, ensure they are of high resolution for better results.
Capabilities
Zero-Shot Generation: Creates stylized portraits without prior training on specific data.
Customization: Allows detailed personalization through prompts and images.
High-Quality Outputs: Produces detailed and aesthetically pleasing images suitable for various applications.
What can I use for?
Artistic Projects: Generate unique couple portraits for digital art, prints, or gifts.
Content Creation: Enhance blogs, social media, or publications with custom imagery.
Design Inspiration: Explore different styles and compositions for creative projects.
Personal Use: Create personalized portraits for special occasions or keepsakes.
Things to be aware of
Historical Styles: Generate portraits in the style of different art movements, such as Baroque or Art Nouveau.
Fantasy Themes: Create imaginative portraits with mythical or sci-fi elements.
Cultural Attire: Produce images featuring traditional clothing from various cultures.
Seasonal Scenes: Design portraits set in different seasons or festive environments.
Limitations
Niche Styles: May struggle with highly specific or obscure artistic styles.
Identity Accuracy: The resemblance to provided identity images might vary, especially with low-quality inputs.
Computational Demand: High-resolution outputs and numerous steps require significant processing power.
Output Format: JPG
Related AI Models
You can seamlessly integrate advanced AI capabilities into your applications without the hassle of managing complex infrastructure.